

In his other older greenhouse, Achim was using HPS lighting, but with 50 to 60 micromol. As far as specialists know, he is the first person in the world to opt for this in his new 2-hectare greenhouse, which was built in 2019.ĭuring the International Propagation Seminar held this autumn, Achim and his crop consultant Hans van Herk of Propagation Solutions talked about their experiences with the lighting supplied by Signify.
#Finetunes distribution full
That is nothing special so far, but the choice for full 100% LED in the nursery is. Komodakis (2016) Wide residual networks.Nowadays, LED lights are installed in the greenhouse of German vegetable plant propagator Achim Gernert of Jungpflanzen Gernert. Feng (2019) Revisit knowledge distillation: a teacher-free framework. Self-training with noisy student improves imagenet classification. Wojna (2016) Rethinking the inception architecture for computer vision. Clune (2015) Deep neural networks are easily fooled: high confidence predictions for unrecognizable images. In Advances in Neural Information Processing Systems, Hinton (2019) When does label smoothing help?.
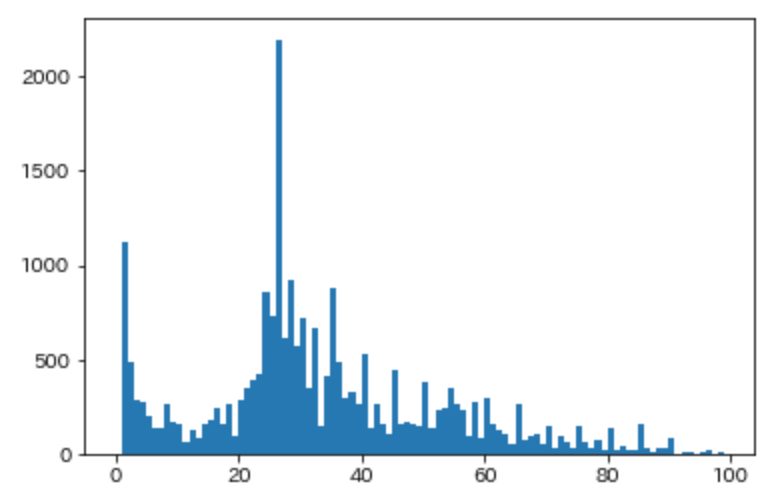
Kumar (2020) Does label smoothing mitigate label noise?. In Proceedings of the IEEE conference on computer vision and pattern recognition,

Weinberger (2017) Densely connected convolutional networks. Dean (2015) Distilling the knowledge in a neural network. Dietterich (2019)ĭeep anomaly detection with outlier exposure. In International Conference on Learning Representations, Gimpel (2017) A baseline for detecting misclassified and out-of-distribution examples in neural networks. In Proceedings of the IEEE Conference on Computer Vision and Pattern Recognition,
#Finetunes distribution how to
Why relu networks yield high-confidence predictions far away from the training data and how to mitigate the problem. Proceedings of the IEEE Conference on Computer Vision and Pattern Recognition, Li (2019)īag of tricks for image classification with convolutional neural networks. In Proceedings of the 34th International Conference on Machine Learning-Volume 70, Weinberger (2017) On calibration of modern neural networks. WRN-40-2 is used as the model architecture of both teacher and student models. (Bottom) teacher models of CIFAR-10 are finetuned with MNIST, TinyImageNet, and MNIST+TinyImageNet by outlier exposure. (Top) teacher models trained with the SVHN, CIFAR-10, and CIFAR-100 dataset and their student models. Figure 2: OOD detection AUROCs of a teacher model and its student model.

When ECE starts to increase (after the red dot line), dramatic drops of AUROC are shown in the training datasets of SVHN (ID) and CIFAR-10 (ID). OOD detection is continuously deteriorated when label smoothing α increases. The red dot line represents label smoothing α minimizing ECE. Figure 1: Test accuracy and expected calibration error (top) and OOD detection AUROC (bottom) of WRN, trained with SVHN (left), CIFAR-10 (middle), and CIFAR-100 (right) respectively. WRN-OE, which is finetuned with TinyImageNet as OOD, is used as the teacher model for the two student models (WRN and DenseNet). Table 2: OOD detection performance of outlier exposure and outlier distillation. OD (Outlier Distillation) means the student model of OE model. TinyImageNet is used to train for OE (Outlier Exposure). Table 1: Test accuracy and expected calibration error (ECE) of WideResNet (Baseline) trained with SVHN, CIFAR10, and CIFAR-100. In addition, we follow the hyper-parameter settings of knowledge distillation in (Müller et al., 2019).įor evaluation of OOD detection, we use the MNIST, Fashion-MNIST, SVHN (or CIFAR-10), LSUN, and TinyImageNet datasets for OOD samples, and AUROC for the evaluation measure.
#Finetunes distribution code
We follow the experimental setting in the official code of outlier exposure 1 1 1 Įxcept that we use 150 epochs for training. In this paper, we train WRN-40-2 (Zagoruyko and Komodakis, 2016) with the SVHN, CIFAR-10, and CIFAR-100 datasets (ID).
